Although it’s a great way to validate your ideas and make data-driven decisions regarding product optimization, it’s not as simple as it sounds.
When running a product experiment, you need to consider feature rollout, troubleshooting, user behavior findings, and decision-making practices while maintaining your product and supporting users that aren’t affected by the recent changes.
Before you even get into this whirlpool, you need to determine whether you need to perform a product experiment at all. Not every idea needs to be tested. Often, releasing a change without a rigorous validation process is the best way to go. So how do you know when product experimentation is required or not?
Throughout this guide, we’ll answer this question and more, including tips on preparing and running experiments, collecting data, and evaluating the results. We’ll also cover the following:
- What is product experimentation?
- When is the right time to run a product experiment?
- 7 Steps to building a robust product experimentation framework
Smartlook records user actions to help you identify opportunities for improvement and evaluate the effectiveness of your experiments. Request a free demo or try Smartlook with a full-featured, 30-day trial: no credit card required.
What is product experimentation?
Product experimentation is the process of testing and trying out variations of a product or feature to see how they perform with users.
Think of it as conducting an experiment in a laboratory, but instead of chemicals and test tubes, you’re experimenting with different aspects, such as design, user interface, features, pricing, and even messaging.
The goal of product experimentation is to collect data to make informed decisions about whether or not you should release a new element at full scale.
Product experimentation allows you to validate your assumptions, test new ideas, and iterate quickly based on user feedback. It’s all about constantly refining and improving your product to deliver the best user experience possible.
3 Benefits of product experimentation for product teams
Product experimentation has a tremendous impact on your product development cycle as it:
- Reduces risk. Product teams can test new ideas on a small scale before investing significant time and resources into a full-scale release. It helps reduce the risk of launching a feature that doesn’t meet the needs of the target audience and hurts the user experience
- Increases innovation. You’ll foster a culture of experimentation and innovation by encouraging teams to explore new ideas and approaches to solving problems in a risk-free environment
- Improves customer knowledge. By regularly testing your hypothesis with real users, you will gain a better understanding of what your customers like and don’t like so you can build a more customer-centric strategy with time
Despite all the significant advantages experimentation brings, it’s not a panacea — sometimes, it’s not necessary. Below, we’ll examine cases when product experimentation is a good idea and when it’s not.
When is the right time to run a product experiment?
Determining the right time is the first step to launching a successful product experiment. It’s very easy to get stuck in a constant loop of experimentation, leading to a strain on your company’s resources.
The right time to run a product experiment depends on several factors, including the stage of product development, the goals of the experiment, and the resources available.
It’s worth stating that a product experiment is a way to validate your ideas with the help of quantitative data. If you don’t have enough users yet, it’s best to focus on qualitative research before you attempt to run experiments. Barada Sahu of Mason suggests hitting at least 10,000 monthly users before you begin experimenting.
Once you have a large enough user base, you can run product experiments whenever you’re considering making significant changes, such as adding new features, changing the pricing model, or redesigning the user interface. These experiments will help you determine whether these changes will be well-received by your users and can help you make data-driven decisions about how to proceed.
No matter what you plan on testing, you should only proceed when the data tells you to do so. Your product team should be constantly monitoring product performance and user behavior to detect issues and spot opportunities for improvement. Only when the data points to customer frustration or hardships can you form a hypothesis regarding what could help fix the problem and run an experiment for validation.
Keep in mind not every idea has to be tested. Say you notice that a lot of people are straying from your user path, failing to complete an intended action. With help from a session replay tool like Smartlook, you might discover they were simply failing to locate the right button. Minor changes like this will fix the issue without requiring you to spend time on experimentation.
There’s no sense in overdoing it with product experiments — the only thing you’ll achieve is wasting time.
A few years ago, Instagram made changes to its interface, switching to a full-screen style feed (apparently, without testing the update with a focus group). After learning that many users resented the new interface, the company reverted back to the original model and “made Instagram normal again.” This story proves that you shouldn’t make changes for the sake of making changes. If something works well, it’s often best to leave it alone.
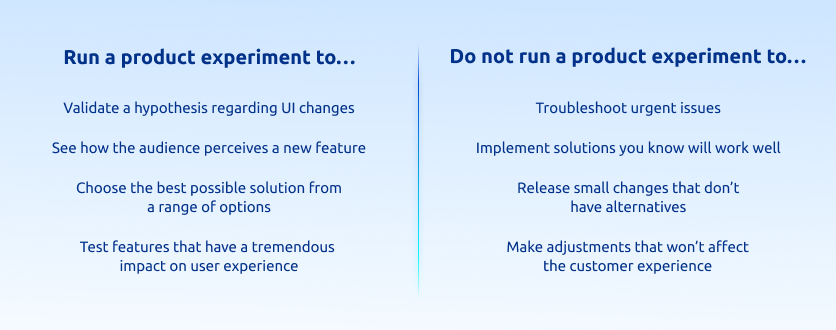
However, if the change is low risk and has a minimal impact on the user experience, it may be appropriate to roll it out immediately to save time and resources. In these cases, we use historical data, common sense, and even predictive analytics to categorize the changes.”
7 steps to building a robust product experimentation framework
Follow these steps to prepare and run a successful product experiment.
1. Look at your product analytics, and spot opportunities for improvement
One doesn’t run an experiment without data at hand. You need to identify a real user problem that needs your attention first. But before you do it, you’ll need to spot problems with your company’s performance first.
In other words, you should start with money.
Is it the customer lifetime value (CLV) that you need to increase? Is it a low activation rate that you need to fix? Look at your top-level performance indicators and focus on one problem at a time.
From here, drill down on product analytics to identify areas that require improvement. Tools like Smartlook can help you track user behavior, pinpointing areas where users are struggling or dropping off.
Start with top-level user engagement metrics and then move on to analyzing user paths with heatmaps and session recordings.
For example, if you are looking to increase your CLV, there’s a good chance you’re dealing with poor customer retention and high churn rates. In this case, you’ll want to identify what’s causing your customers to feel frustrated with your product. To answer this question, it’s best to ask them about it. With exit surveys, you’ll determine what aspects of your product aren’t meeting user expectations. You can then turn to session recordings to see how users are interacting with the features they reported they liked least. From here, you can make assumptions regarding how you can improve your product’s functionality or UI.
You can also run in-app surveys from Survicate that ask active users to rate specific features. This way, you’ll collect valuable quantitative insights that highlight areas that require special attention. By integrating Survicate with your Smartlook account, you can drill down into the data by viewing the session recordings behind each response.
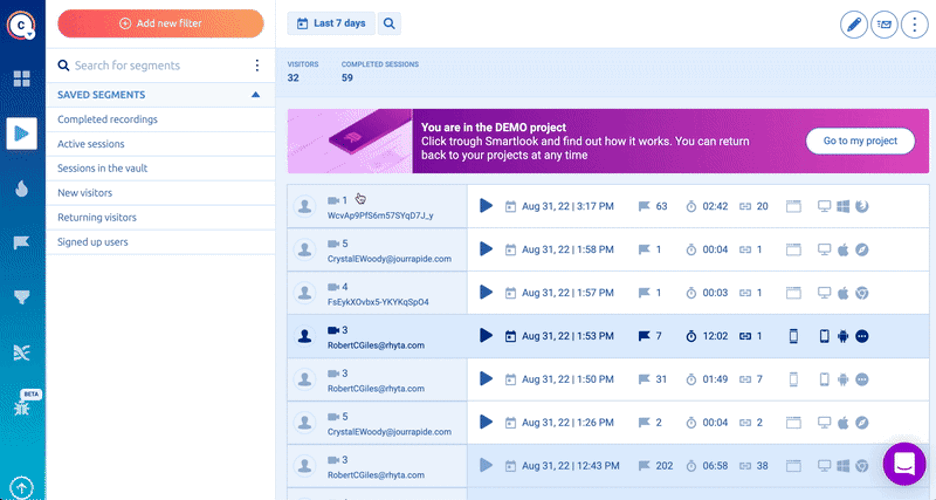
2. Develop a hypothesis
Once you’ve identified areas for improvement, it’s time to develop a hypothesis relating to optimizing the product experience. This hypothesis should be based on your understanding of the problem your users are facing and should clearly state how you plan to address it.
For example, if your CLV is low because users are having trouble using your product, you could hypothesize that by adding interactive product walkthroughs, you’ll improve retention and ultimately increase CLV. Just make sure your hypothesis is specific, measurable, and testable.
3. Choose a testing model
How exactly are you going to run an experiment? There are several testing models to choose from, including:
- A/B testing. This involves creating two versions of a product and randomly assigning users to one or the other. Once the test is complete, compare the performance of each version to see which performs best
- Multivariate testing. Instead of implementing one change at a time, change multiple variables at once. Multivariate testing allows you to test various combinations to see which performs best
- Funnel testing. This type of product experimentation involves making changes to different product pages to create and test new user paths. This is a perfect experimentation technique when you need to adjust the entire user flow but want to test it first
Smartlook helps you validate your experiments. Simply set up your variations in Firebase, Google Optimize, or another product testing tool and specify your variation IDs in Smartlook. You can then group sessions based on experiment and variation IDs and evaluate the impact of your tests using funnels and events.
That said, there are a lot of different ways you can do this. For example, A/B tests are great because they let you compare two different versions of something (like button color and font) and see which one performs better.
But we don’t think any one technique will work for every product team. It really depends on what kind of product you’re trying to build, your goals for testing, etc.
One thing we always recommend is using real users as part of the testing process. This way, you’ll be able to get feedback from the people who actually use your product — not just from yourself or other members of your team!”
4. Define KPIs for your test
To measure the success of your product experiments, you’ll need to specify your KPIs early. Consider the following criteria when defining your experimentation KPIs:
- Alignment with goals: The KPIs you choose should align with your experimentation goals. For example, if you’re testing a new onboarding flow, your KPIs might include metrics such as the percentage of users who complete the onboarding process, the time it takes to complete the process, and the drop-off rate
- Focus on user behavior: Your KPIs should focus on user behavior in addition to metrics like pageviews and session length. User behavior metrics will help you understand how users interact with your product and provide insights into how you can improve the user experience
- Set benchmarks: It’s important to set benchmarks for your KPIs so you can measure progress over time. This will help you understand whether your experiments are having an impact
Once you know which metrics will help you evaluate your experiment results, you can set up tracking.
5. Set up tracking
To do this, you’ll need to choose a tracking tool. Select a tool that supports the metrics you want to track and the testing method you’ve chosen. Tools like Firebase and Google Optimize support different experimentation forms and deliver insightful reports on the performance of your tests.
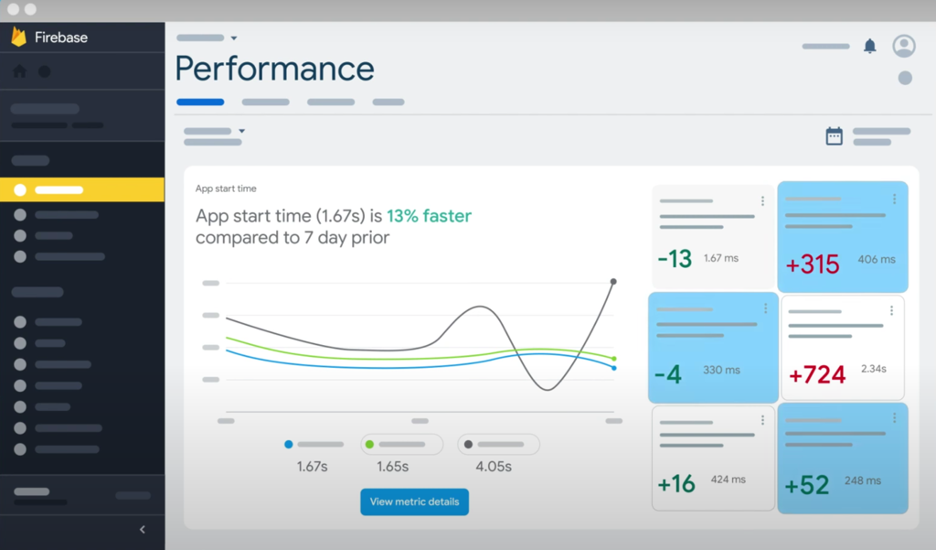
Monitoring test performance with Firebase.
Consider adding another layer of qualitative insight to your experiments. While your experimentation tool will help you determine a winning version of your product, there should be a visual insights tool that will help you understand the reasons behind the results. For instance, if you choose to run tests with Firebase, integrating it with a tool like Smartlook will help you get more granular insights into how users are responding to the changes.
Next, add the tracking code and set up events and goals to start collecting data. This will allow you to track specific user actions, such as clicking a button or filling out a form.
Test your tracking to ensure it’s working properly, and validate your data to make sure it’s accurate.
6. Determine what you consider to be statistically significant results
How do you know if your test results are significant enough to make conclusions on the effectiveness of the experiment? The short answer is that you’ll need to determine testing parameters beforehand.
Testing parameters include audience sample size and the length of the experiment required to achieve statistically significant results.
Statistical significance is a measure of whether the difference between two variations is real or simply due to chance. For example, if you try to test a new UI against ten users split into two groups, the results won’t be statistically significant due to the small size of the sample audience.
The larger the audience sample size and the longer the test lasts, the more reliable the results your experiment will deliver.
The significance level or alpha indicates the probability that the observed difference between groups is due to chance. If the change is higher than alpha, it is statistically significant. The bigger the difference, the better.”
7. Run the experiment and evaluate the results
When you’re all set, you can launch your experiment. Don’t wait for the test to end to monitor its performance — keep an eye on the metrics all the time to spot issues as they arise.
After the test is over, collect and analyze the data to determine whether the experiment was successful or not. Your experimentation tool will provide you with a clear verdict on which product version won — this is where you discover whether your hypothesis is confirmed or not.
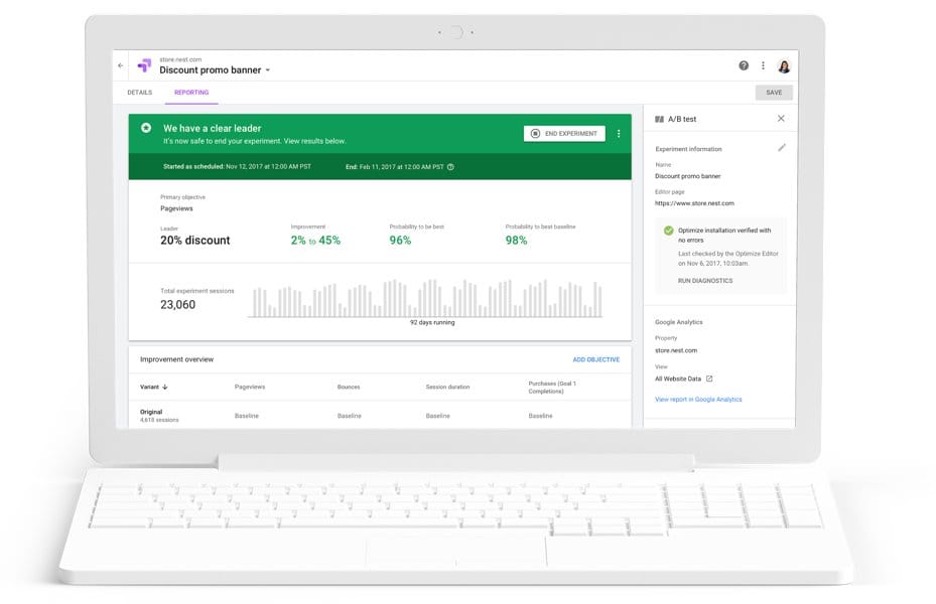
Defining a winning variant with Google Optimize.
Regardless of the verdict, go beyond the metrics and look into the behaviors users exhibited during the experiment. Watch how they interacted with new features or workflows to spot moments of frustration or positive experiences.
Document the results once you’ve finished your analysis. Share your findings with your team and stakeholders. This will help you create more successful experiments in the future.
Remember, you can’t improve everyone’s experience. If you see a significant improvement in KPIs after the test, it’s a win — even though some users may be failing to accept the change.
Run data-driven product experiments with Smartlook
Product experimentation is an easy way to validate your ideas for improvement. That said, it can be tricky. Overdo it, and you’ll waste time and money instead of quickly making necessary adjustments. Underdo it, and you’ll waste time and money releasing features users don’t need.
The best way to understand when to run new product experimentation is by looking into your product data. Smartlook will provide you with quantitative and qualitative insights to not only spot opportunities for improvement but also to figure out whether the improvements require a rigorous experimentation process or not.
Furthermore, Smartlook helps you evaluate the effectiveness of your experiments with granular user behavior insights. For example, you can set up events to see how your test group interacts with a new feature or monitor heatmaps to see how a UI change affects the user experience.
To learn more about how Smartlook can help you with product experimentation, schedule a free demo with our team today. You can also try Smartlook for free with a full-featured, 30-day trial.